R in Insurance, London 2013
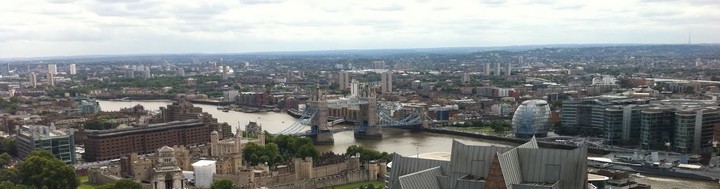
The first R in Insurance conference took place at Cass Business School, London, 15 July 2013.
Programme
- 9:00 - 10:00 Opening Keynote
- Implementing CreditRisk+ in R with the Faster Fourier Transform - Professor Alexander McNeil [slides]
- 10:00 - 11:00 Contributed Talks
- A practical approach to claims reserving using state space models with growth curves - Chibisi Chima-Okereke [slides]
- A new R-package for statistical modelling and forecasting in non-life insurance - Lola Martínez-Miranda [slides]
- A re-reserving algorithm to derive the 1-year reserve risk view - Alessandro Carrato [slides]
- 11:00 - 11:30 Tea/Coffee
- 11:30 - 12:30 Contributed Talks
- 12:30 - 13:30 Lunch
- 13:30 - 14:30 Contributed Talks
- End User Computing: Excel / VBA vs. R - Karen Seidel and Richard Pugh
- Claim fraud analytics with R - Enzo Martoglio and Adam Green
- Integrating R with Azure for High-throughput analysis - Hugh P. Shanahan [slides]
- 14:30 - 15:00 Panel discussion
- The Future of R in Insurance
- 15:00 - 15:30 Tea/Coffee
- 15:30 - 16:30 Contributed Talks
- Automate presentations of management information with R - Simon Brickman and Adam Rich
- Practical implementation of R in the London Market - Ed Tredger and Fiachra McLoughlin
- Catastrophe Modelling in R - Stefan Eppert [slides]
- 16:30 - 17:30 Closing Keynote
- There is an R in Lloyd’s - Trevor Maynard [slides]
- 17:30 - 18:30 Drinks reception
- 18:45 Bus transfer to conference dinner restaurant
- 19:00 Conference dinner at Cantina del Ponte which lies on the banks of the Thames, over-looking Tower Bridge
Abstracts
Implementing CreditRisk+ in R with the Faster Fourier Transform
Professor Alexander McNeil
Department of Actuarial Science & Statistics, Heriot-Watt University
The well-known CreditRisk+ model of portfolio credit risk is often described as “an actuarial model”. Conditional on independent gamma-distributed economic factors, credit losses in fixed time periods are conditionally independent Poisson events. Exposures are usually discretised into a finite number of exposure bands. This leads to a reasonably tractable model that can be represented in terms of compound sums.
We will review the structure of the model and then show how it can be easily implemented in R. We focus on computing the portfolio loss distribution using Fourier inversion techniques and deriving measures of tail risk. We will also discuss the calibration of the model.
A practical approach to claims reserving using state space models with growth curves
Chibisi Chima-Okereke
Active Analytics Ltd
State space models offer much flexibility in dealing with general time series and regression problems. Bayesian approach means that expert judgement can be used in their formulation and they offer the benefit of allowing the modeller to use information available at any time period to pre-empt the effects of expected changes or increased uncertainty in forecasts rather than being limited by more classical approaches. This makes them valuable for many applications and they are considered here for the calculation of actuarial reserves.
In this talk, a state space model using various growth curves for modelling claims developments is presented. These curves are used to model logarithm and inverse transformed cumulative claims as well as development patterns. An advantage of the state space modelling procedure is that a standard output of the model are parametric ultimate claims forecast distributions for state and observations. The parameters used in the state matrix are obtained from no-linear regression of curves from the claims triangle.
Intervention techniques allow the modeller to quickly asses the effects of new information before subsequent observations are obtained. The model can also be used as a tool for pre-empting the effects of potentially large claim events on the business class or increased uncertainty in the underwriting environment.
This technique is compared with outputs from the chain ladder method. The models are created using R, a rich statistical analysis environment which also provides a framework for creating space state models as well as allowing the user to create custom algorithms.
A new R-package for statistical modelling and forecasting in non-life insurance
Martínez-Miranda, M.D., Nielsen, J.P. and Verrall, R.
Cass Business School
The recent Double Chain Ladder (DCL) by Martínez-Miranda, Nielsen and Verrall (2012) has demonstrated how the classical chain ladder technique can be broken down into its components. It was shown that DCL works under a wide array of stochastic assumptions on the nature and dependency structure of payments. Under certain model assumptions and via one particular estimation technique, it is possible to interpret the classical chain ladder method as a model of the observed number of counts with a build-in delay function from a claim is reported until it is paid. Under the DCL framework it is possible to gain a deeper understanding of the fundamental drivers of the claims development than is possible with the basic chain ladder technique. One example is the case when expert knowledge is available and one would like to incorporate it into the statistical analysis. This can be done in a surprisingly simple way to include into a double chain ladder framework.
In this talk we present a new package in R to analyse run-off triangles in the double chain ladder framework. The package, which is expected to be launched in July 2013, contains several functions to assist the user along the full reserving exercise. Using specific functions in the package the user will be able to load the data into R from Excel spreadsheets, make the necessary manipulations on the data, generate plots to visualize and gain intuition about the data, break down classical chain ladder under the DCL model, visualize the underlying delay function and the inflation, introduce expert knowledge about the severity inflation, the zero-claims etc. The package contains also data examples and has been documented to facilitate the analyses to a wide audience, which includes practitioners, academic researchers and also undergraduate, master and PhD students. Using the package the user will be able to reproduce the methodology of the recent papers by Martínez-Miranda, Nielsen, Nielsen and Verrall (2011), Martínez-Miranda, Nielsen and Verrall (2012, 2013), Martínez-Miranda, Nielsen and Wüthrich (2012) and Martínez-Miranda, Nielsen, Verrall and Wüthrich (2013).
References:
- Martinez-Miranda M.D, Nielsen B, Nielsen J.P and Verrall, R. (2011) “Cash flow simulation for a model of outstanding liabilities based on claim amounts and claim numbers”. Astin Bulletin, 41/1, 107-129.
- Martínez-Miranda, M.D., Nielsen, J.P. and Verrall, R. (2012) “Double Chain Ladder”. Astin Bulletin, 42/1, 59-76.
- Martínez-Miranda, M.D., Nielsen, J.P. and Verrall, R. (2013) “Double Chain Ladder and Bornhuetter-Ferguson”. North American Actuarial Journal.
- Martínez-Miranda, M.D., Nielsen, J.P. and Wüthrich, M.V. (2012) “Statistical modelling and forecasting in Non-life insurance”. SORT-Statistics and Operations Research Transactions 36 (2) July-December 2012, 195-218.
- Martínez-Miranda, M.D., Nielsen, J.P., Verrall, R. and Wüthrich, M.V. (2013) “Double Chain Ladder, Claims Development Inflation and Zero Claims”. Scandinavian Actuarial Journal.
A re-reserving algorithm to derive the 1-year reserve risk view
Alessandro Carrato1
1Fully Qualified Actuary, Istituto Italiano degli Attuari / International Actuarial Association - ASTIN Section
Keywords: reserve risk, one-year view, re-reserving, ultimate view, model error, solvency 2
I consider a practical approach, based on R code, to the methodology for the one-year view reserve risk described by [1]. The idea is to extend the re-reserving algorithm outside the chain ladder model (see [2]), introducing a proper algorithm that works directly on the underlying GLM model defined for the ultimate view, and updated with the simulated payments after 1 year. Besides, the R code gives also the option to change the regression structure, distribution in the exponential family and link function of the ultimate-view reserve risk (see [3] and [4]) in order to permit a better understanding and evaluation of the model error, as required by Solvency 2 (see [5]).
References
- Ohlsson et al. (2008) – The one-year non life insurance risk [ASTIN Colloquia 2008]
- Merz, Wüthrich (2008) – Modelling CDR for Solvency purposes [CAS E-Forum, Fall 2008, 542-568]
- Gigante, Sigalotti (2005) – Model Risk In Claims Reserving with GLM [Giornale Istituto Italiano degli Attuari LXVIII, n. 1-2, pp. 55-87, 0390-5780]
- Wüthrich, Merz (2008) – Stochastic Claims Reserving Methods in Insurance [The Wiley Finance Series]
- EIOPA (2012) – Technical Specifications for the Solvency II valuation and Solvency Capital Requirements calculations [SCR 1.23, p. 119]
Pricing insurance contracts with R
Giorgio Alfredo Spedicato Ph.D C.Stat ACAS
The R statistical system [3] could be a very powerful tool to price contracts in the business of insurance. As 2013, several packages already exist that can aid pricing actuaries in their activity. This presentation will show how standard R code enhanced by ad - hoc packages could provide sound actuarial solutions for real business.
A first example could be pricing life contingent coverages for life insurance business. Few examples performed with the aid of lifecontingencies package [5] will show how R can be easily used to perform standard pricing and reserving for life insurances.
A second set of examples will show how GLM estimation capabilities of R statistical environment can be used to perform standard pricing of personal lines general insurance coverages. Examples will be taken from [4] working paper.
The last set of example briefly show an application of actuar [2] and fitdistrplus [1] packages to price non-proportional reinsurance coverage for a Motor Third Party Liability portfolio.
References
- Marie Laure Delignette-Muller, Regis Pouillot, Jean-Baptiste Denis, and Christophe Dutang. fitdistrplus: help to fit of a parametric distribution to non-censored or censored data, 2012. R package version 1.0-0.
- Christophe Dutang, Vincent Goulet, and Mathieu Pigeon. actuar: An r package for actuarial science. Journal of Statistical Software, 25(7):38, 2008.
- R Development Core Team. R: A Language and Environment for Statistical Computing. R Foundation for Statistical Computing, Vienna, Austria, 2012. ISBN 3-900051-07-0.
- Giorgio Alfredo Spedicato. Third party motor liability ratemak- ing with R. 6 2012. Casualty Actuarial Society Working Paper.
- Giorgio Alfredo Spedicato. Lifecontingencies: an R package to perform life contingencies actuarial mathematics, 02 2013. R package version 0.9.7.
Mortality modelling in R: an analysis of mortality trends by cause of death and socio-economic circumstances in England
Andrés M. Villegas
Cass Business School
Keywords: Mortality modelling; Lee-Carter model; socio-economic circumstances; cause of death; ggplot2; gnm; forecast.
It is well known that mortality rates and life expectancy vary across socio-economic subpopulations of a country. Higher socio-economic groups - whether defined by educational attainment, occupation, income or area deprivation - have lower mortality rates and longer lives than lower socio-economic groups. In many cases, high socio-economic subpopulations also experience faster rates of improvement in mortality. These socio-economic differences pose important challenges when designing public policies for tackling social inequalities, as well as when managing the longevity risk in pension funds and annuity portfolios. The successful addressing of these social and financial challenges requires the best possible understanding of what has happened historically and what is likely to occur in the future. A key step in this direction is to investigate how individual causes of death differ between the different socio-economic subgroups of the population.
In this talk we illustrate how R can be used in the analysis of recent trends in mortality by cause of death and socio-economic stratification, using mortality data for England split by socio-economic circumstances. More specifically, we demonstrate how existing R packages can be used in the preliminary analysis and visualisation of mortality data (ggplot2) and in the modelling (gnm) and projection (forecast) of mortality trends employing multi-population extensions of the popular Lee-Carter mortality model.
References
- Hyndman, R. J, 2013. forecast: Forecasting functions for time series. R package version 4.03.
- Turner, H., Firth, D., 2012. Generalized nonlinear models in R: an overview of the gnm package. R Package Version 1.0-6.
- Wickham, H., 2009. ggplot2: elegant graphics for data analysis. Springer New York.
Non-Life Insurance Pricing using R
Allan Engelhardt and Suresh Gangam
CYBAEA Limited, London, UK and 64 Squares, Maharashtra, India
Insurance can greatly benefit from adopting the R platform and leading companies are already reaping the rewards. We will show one example from non-life insurance pricing which will cover both technical implementation and business change, and we will share information on the commercial benefits obtained. By using a specific example we can keep the presentation concrete and the benefits real; however, the applicability of the approach is general and we will touch on this in the discussion.
There are many advantages of R. We will focus on two. First, R is finely balanced to allow exploratory data analysis and interactive model development while also being a platform for statistical computing and data mining. As we will show, this is key for productivity and an element to set up (bit-perfect) reproducible models.
Second, it is comprehensive in the sense that most approaches to statistics and data mining are included in the tool or its contributed packages. Among other benefits, this allows you to easily run multiple model types on your data, ensuring compatibility with classic and often robust approaches while at the same time taking advantage of the latest developments and emerging industry standards.
Non-life insurance pricing is a well-known and well-established process and yet still a critical business issue. The standard for tariff analysis is generalised linear models. We first show how to develop such a model in R, including model selection and validation. We touch upon how to deploy the model (both scoring using the model and updating the model itself) while ensuring the results remain validated and reproducible.
Next we show how easy it is to extend the model to more complex techniques. In the interest of time we jump over intermediate approaches and go straight to ensemble models, which are possibly the state-of-the-art for high-performance models.
We are in no way advocating wholesale abandonment of classical approaches for modern techniques, “black-box” or otherwise. Rather, we propose that you make use of both: continuity and understanding tempered with the results from the latest up-to-date methods. In the final part we cover some of these business issues to show how other insurers resolved them and what commercial benefits resulted. Examples include using the advanced models to restrict the validity domain of the classical approach (risk we do not understand and will not insure) and using them to create derived variables, such as interaction variables, to extend the domain of the GLM (understanding complex risk).
End User Computing: Excel / VBA vs. R
Karen Seidel and Richard Pugh
Lloyd’s and Mango Solutions
Most actuarial departments in the Non-Life insurance industry use Excel /VBA as their computation engine. Industry leading bespoke modelling software, such as Igloo and ReMetrica relies on Excel / VBA for data inputs and reporting. This talk points out the typical problems that arise from using Excel / VBA in capital modelling and how these issues can be overcome with a combination of R and a proper version control system. Issues covered include
- Keeping track of links
- Keeping track of different versions of input data, model code and outputs
- Support for multiple users
- Trickiness of updates (eg range adjustments for a new underwriting year)
- Limitations of Excel analyses
- Limitations of reporting in Excel
- Constraints on data volumes
Claim fraud analytics with R
Enzo Martoglio and Adam Green
Steria UK and Syntomy
According to different sources the insurance sector is plagued by fraudulent claims: in the UK alone total undetected general insurance claims fraud is estimated at £1.9 billion per annum. This adds around 6% (or £44 a year), on average, to the insurance premiums paid by all policyholders (Research Brief - 2009 Association of British Insurers).
R offer powerful analytical functions to detect fraudulent claims. They range from network analysis, typically used to monitor fraudulent motoring claims, to text analytics.
The presentation aims to:
- Offer a brief overview of the R packages that can be used for fraudulent claim analytics (e.g. how network analytics can be used to spot frauds etc.).
- Illustrate the analytical pipeline component required to detect potentially fraudulent claims using text analytics. One of the components illustrated will be the use of the LIWC (Linguistic Inquiry and Word Count) dictionary.
- Link claims with the general insurance process to show the benefits obtained through a wider usage of analytics.
Please note that currently we plan to illustrate the above using dummy data, as any insurance company is reluctant to “loan” their data for analysis.
Integrating R with Azure for High-throughput analysis
Hugh P. Shanahan1, Anne M Owen2, Andrew P. Harrison3
1Department of Computer Science, Royal Holloway, University of London, Egham, Surrey, TW20 0EX, U.K., 2Department of Mathematical Sciences, University of Essex, Wivenhoe Park, Colchester, CO4 3SQ, U.K., 3Department of Biological Sciences, University of Essex, Wivenhoe Park, Colchester, CO4 3SQ, U.K.
Keywords: Cloud Computing, Azure, PaaS, High-throughput
Cloud Computing is increasingly being used by the Scientific community. For example, in Bionformatics this has been largely driven by the rapid increase in the size of Omic (Genomic, Transcriptomic,…) data sets Stein (2010). This rapid increase in data size is not unique to this field and is a surprisingly general feature in data analysis. This type of computing is particularly useful for a workflow where one needs to execute a complicated analysis (e.g. a large R script) in a trivially parallel fashion over a large data set. Within Insurance possible applications for such high-throughput calculations include
- time-series analysis which require extensive parameter sweeps or
- VaR calculations for a portfolio of a large number of various financial instruments Kim (2009).
Much of the emphasis in cloud computing has been on the use of Infrastructure as a Service platforms, such as Amazon’s EC2 service where the user gets direct access to the console of the Virtual Machines(VM’s) and MapReduce frameworks, in particular Hadoop Yoo (2011). An alternative to this is to use a Platform as a Service (PaaS) infrastructure, where access to the VM’s is programmatic. Other PaaS clouds exists, notably the Google App Engine but are limited due to a conservative approach to allowing libraries on the App Engine.
A PaaS interface can offer certain advantages over the other approaches. In particular, it is more straight- forward to design interfaces to software packages such as R. In the case of Azure, another advantage is that Microsoft Research have provided a set of C# libraries called the Generic Worker which allow easy scaling of VM’s.
We have developed software that makes use of these libraries to run R scripts to analyse a particular data set approximately 1 Tbyte in total size though decomposed into a number of a much smaller units. This analysis provides an exemplar to run multiple R jobs in parallel with each other on the Azure platform and to make use of its mass storage facilities. We believe that this workflow is a very common one and is applicable to any number of different areas where R is employed. We will discuss an early generalisation we have dubbed GWydiR to run any R script on Azure in this fashion, with a goal on providing as simple a method as possible for a user to scale up their R jobs.
References
- Stein, L. D. (2010, January). The case for cloud computing in genome informatics. Genome biology 11(5), 207.
- Hyunjoo, K., Chaudhari, S., Parashar, M. and Marty, C. (2009) Online Risk Analytics on the Cloud. 9th IEEE/ACM International Symposium on Cluster Computing and the Grid, 2009. CCGRID ’09 484-489 DOI:0.1109/CCGRID.2009.82
- Yoo, D. and Sim, K-M. (2011). A comparative review of job scheduling for MapReduce., 2011 IEEE International Conference on Cloud Computing and Intelligence Systems (CCIS) 353-358. DOI:10.1109/CCIS.2011.6045089
Automate presentations of management information with R
Simon Brickman and Adam Rich
Beazley
The talk will provide R code to show how to automate the presentation of key charts, tables and reports.
This will be in the context of providing information to general insurance professionals who are mainly non actuarial. Typical audience is underwriters and claims managers. The goal here is to impart the maximum clarity to the information whilst also making the production task easy and flexible.
The code used will essentially comprise of existing package material: the intellectual added value provided here is really around the collation of this material into a useful bundle of value to analytical practitioners. The talk will also compare and contrast the process with current alternatives used in the industry and discuss ideas for future development to assist actuaries in their roles within general insurance.
Practical implementation of R in the London Market
Ed Tredger and Fiachra McLoughlin
UMACS
Our talk will focus on the massive potential for R in the London Insurance Market, our practical experiences of using it with our insurance clients and the main obstacles R faces in gaining wider acceptance and usage in the London Market. All of this talk is based on practical experience of using R in real-world examples and draws from the presenter’s personal experience.
There are three distinct sections to the talk:
- Why R is useful in the London market
- Personal experiences of using R in real-world problems
- Practical barriers to using R in Insurance
Since the first part of the talk will be well-understood by most attendees, this will be the briefest, but will offer our perspective based on the model development and modelling projects we deliver across a wide range of Lloyd’s and London Market clients.
The second part will discuss different applications of R we have found useful, how they have been implemented and what value they have added to the client. This part of the talk will use examples of how R has been successfully used in pricing, reporting and in producing Lloyd’s returns.
The third part of the talk is likely to prompt the most discussion; here we will discuss the barriers R encounters in Insurance and how these might be overcome. There is little doubt that while seasoned R users believe strongly in its abilities R has not, yet, reached a high level of market penetration. We hope that this talk will stimulate debate within the audience about overcoming these obstacles so that R can achieve wider recognition throughout the Insurance industry.
Catastrophe Modelling in R
S. Eppert, D. Lohmann and G. Morrow
KatRisk LLC, 405 Kains Ave, Albany CA 94706, USA
Catastrophe (cat) models are used to estimate loss distributions from natural hazards like tropical cyclones, floods, or earthquakes. They integrate multiple disciplines such as meteorology, climatology, hydrology, structural engineering, statistics, software engineering and actuarial sciences.
The ever increasing complexity of these models, the need for model transparency, as well as the desire to integrate models with diverse APIs have led us to develop an open source web-based cat model engine based on R using Shiny.
By using R, users can easily create custom analytics and integrate auxiliary data from any data source, while being able to probe underlying model assumptions, perform sensitivity analysis and in- vestigate all components of the cat model. We will demo our software and speak about the various technology components.
There is an R in Lloyd’s
Trevor Maynard
Head of Exposure Management and Reinsurance, Lloyd’s
In 2005, a group of nerds in Lloyd’s (with one honorary member from outside) started a group called R Souls (say it fast and you’ll get the joke).
They met every Friday to make the most of the fish and chips and swapped stories about R; learning from one another and becoming ever more proficient in the amazingly stable, flexible and exciting tool that is, R.
From these humble beginnings R is now embedded in many of Lloyd’s core functions from benchmarking and reporting to catastrophe modelling.
My talk will give a short history of this turbulent and emotional journey including some tips on how to work with IT departments, and convince others to move from planet Excel to the 21st century.
Sponsors
- Mango Solutions
- CYBAEA